Healthcare AI systems that put people at the center
Over the past four years, Google has advanced its AI technologies to address critical problems in healthcare. We’ve developed tools to detect eye disease, AI systems to identify cardiovascular risk factors and signs of anemia, and to improve breast cancer screening.
For these and other AI healthcare applications, the journey from initial research to useful product can take years. One part of that journey is conducting user-centered research. Applied to healthcare, this type of research means studying how care is delivered and how it benefits patients, so we can better understand how algorithms could help, or even inadvertently hinder, assessment and diagnosis.
Our research in practice
For our latest research paper, "A Human-Centered Evaluation of a Deep Learning System Deployed in Clinics for the Detection of Diabetic Retinopathy," we built on a partnership with the Ministry of Public Health in Thailand to conduct field research in clinics across the provinces of Pathum Thani and Chiang Mai. It’s one of the first published studies examining how a deep learning system is used in patient care, and it’s the first study of its kind that looks at how nurses use an AI system to screen patients for diabetic retinopathy.
Over a period of eight months, we made regular visits to 11 clinics. At each clinic, we observed how diabetes nurses handle eye screenings, and we interviewed them to understand how to refine this technology. We did our field research alongside a study to evaluate the feasibility and performance of the deep learning system in the clinic, with patients who agreed to be carefully observed and medically supervised during the study.
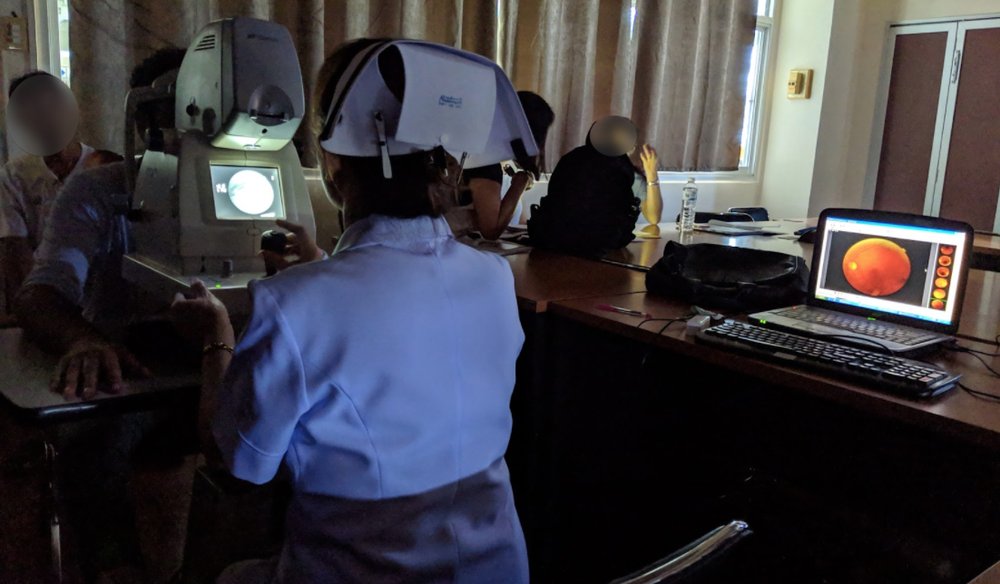
A nurse operates the fundus camera, taking images of a patient’s retina.
The observational process
In our research, we provide key recommendations for continued product development, and provide guidance on deploying AI in real-world scenarios for other research projects.
Developing new products with a user-centered design process requires involving the people who would interact with the technology early in development. This means getting a deep understanding of people’s needs, expectations, values and preferences, and testing ideas and prototypes with them throughout the entire process. When it comes to AI systems in healthcare, we pay special attention to the healthcare environment, current workflows, system transparency, and trust.
The impact of environment on AI
In addition to these factors, our fieldwork found that we must also factor in environmental differences like lighting, which vary among clinics and can impact the quality of images. Just as an experienced clinician might know how to account for these variables in order to assess it, AI systems also need to be trained to handle these situations.
For instance, some images captured in screening might have issues like blurs or dark areas. An AI system might conservatively call some of these images “ungradable” because the issues might obscure critical anatomical features that are required to provide a definitive result. For clinicians, the gradability of an image may vary depending on one’s own clinical set-up or experience. Building an AI tool to accommodate this spectrum is a challenge, as any disagreements between the system and the clinician can lead to frustration. In response to our observations, we amended the research protocol to have eye specialists review such ungradable images alongside the patient’s medical records, instead of automatically referring patients with ungradable images to an ophthalmologist. This helped to ensure a referral was necessary, and reduced unnecessary travel, missed work, and anxiety about receiving a possible false positive result.
Finally, alongside evaluating the performance, reliability, and clinical safety of an AI system, the study also accounts for the human impacts of integrating an AI system into patient care. For example, the study found that the AI system could empower nurses to confidently and immediately identify a positive screening, resulting in quicker referrals to an ophthalmologist.
So what does all of this mean?
Deploying an AI system by considering a diverse set of perspectives in the design and development process is just one part of introducing new health technology that requires human interaction. It's important to also study and incorporate real-life evaluations in the clinic, and engage meaningfully with clinicians and patients, before the technology is widely deployed. That’s how we can best inform improvements to the technology, and how it is integrated into care, to meet the needs of clinicians and patients.
by Emma Beede via The Keyword
Comments
Post a Comment